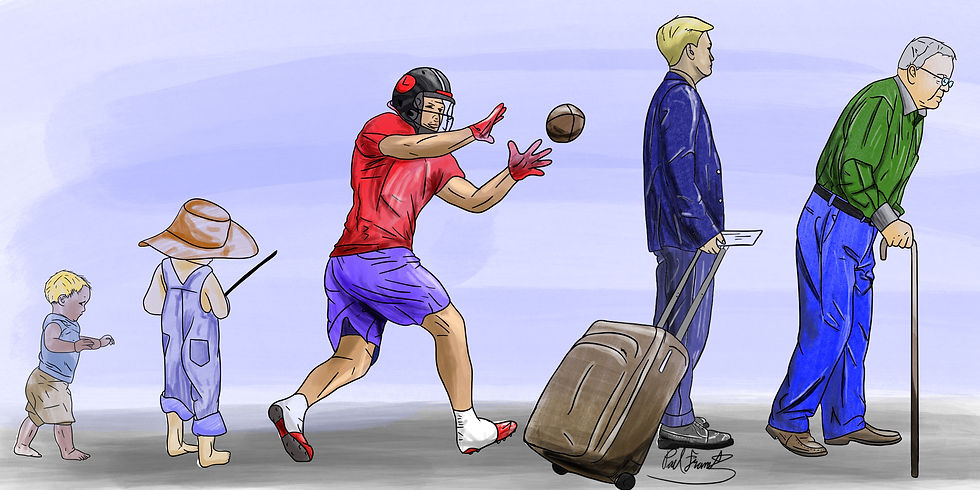
“Forecasting the Future” is this month’s artistic interpretation by Paul Frantz of the Ask the Experts discussion. How accurate are you with predicting the course of your own life? Looking back at your life, the future uncertainties would have been better described by ranges and not by point estimates. The future is, at best, a wide interstate highway and not a narrow path.
This month’s Ask the Experts is a one-on-one lightning round with Gustavo Vinueza. Gustavo has extensive, international experience in forecasting, uncertainty analysis, and simulations. As a longtime portfolio manager, custom solutions leader, and technical support specialist for Palisade Corporation, makers of the DecisionTools Suite and @Risk, Gustavo has worked for many years with a wide variety of companies and their executives in the decision-making space, helping to anticipate trends and borderline situations. He is a frequent speaker in forums on several continents related to uncertainty and simulation. Gustavo has an undergraduate degree in systems engineering and master’s degrees in both business administration and finance.
We were pleased to have Seth Robertson moderate the conversation. Seth is an accomplished engineer, project manager, and program administrator who currently leads the finance & asset management practice for a Raleigh-based consulting firm. As the former state section chief of the entity that provides infrastructure grants and loans to units of local government, Seth has experienced the good and the bad of forecasts developed by owners and their consultants.
Forecasting is an expansive topic that is essential to solving important and unusual problems. Several important themes emerged from the session and follow-up discussions. First, there are more than one method to developing forecasts and we often spend too much time arguing about the best method. Second, Monte Carlo analysis is a cheap, fast, and simple method for gaining important insights. Third, uncertainty should be embraced because it is reality. And fourth, we do not do enough back-testing and post-mortems of the forecasts we make.
How important is understanding “cause and effect” prior to building a model to forecast the future? Can we simply look for trends in a past data set?
JD: All good models start from understanding the handful of primary causes that drive an answer.
GV: Cause and effect is fundamental. A quick victory is to start with a detailed examination of your data to see if it makes sense.
JD: From the school of hard knocks, I have had my share of experience in diving into the model and the forecast too quickly under the assumption that the data was valid. Later I found out that the data was not valid or that we did not have the data that supported cause and effect.
GV: A practical tip is to examine the data with a correlation analysis. You will gain some insights as to whether the data trends in a manner consistent with your understanding of cause and effect.
What is the toughest part of forecasting an uncertain future?
GV: Explaining the results to the customer
JD: I expected you to say something about the data or technical analysis. That answer really surprises me.
GV: Validating the models would be my second toughest part, but it is a distant second. The first one is the one JD has mastered – explaining to the customer.
JD: For me, the toughest part is validation because we often do not have the money on the back end to validate results or to validate the inputs, we modeled are truly causal. We mentioned my next aspect that I find toughest, which is understanding cause and effect before we build our model.
Are using point estimates for input parameters the best way to do forecasting?
JD: No, we tend to miss widely when we use single-point estimates to forecast.
GV: To me, the point estimate is best for what we call version zero. Maybe it is a start, but you must improve it.
JD: With that said, I do not think Monte Carlo analysis is relevant for every forecast. It really depends on the context.
GV: For some types of problems, you may be in the ballpark with high, medium, and low estimates of input parameters. Forecasts based on a single point estimate for each parameter are science fiction.
What is the biggest advantage of using probabilistic analysis, such as Monte Carlo analysis, for forecasting?
GV: In a simple way it allows you to explore uncertainty. It forces you to start thinking in ranges.
JD: Thinking in ranges and explaining in ranges. In other words, you can shift the conversation from “can you make this budget” to “if you give me that much budget, this is our chance of success”
GV: It’s a little bit of an argument with people who are very mathematical and like big equations, but Monte Carlo gives us simple models in minutes rather than weeks or months.
JD: That may be good for the modeler but what about the decision-maker?
GV: It is a tricky question. If we simplify too much, then we underfit and our forecasts are bad. We have to have some standards established up front as to what is acceptable and what is not. And that is true for any type of model.
What is the biggest disadvantage of Monte Carlo analysis?
JD: Mathematically and statistically, people get wrapped around the axle and worry too much about where and how the distributions came from. It is important to get started and make sure you add more examination as you gain initial insights.
GV: Not paying attention to the distributions is a recipe for disaster. It is so easy to be wrong and the intuitions will look good enough. People can go for years and not realize they are wrong.
JD: My second disadvantage of Monte Carlo is continually having to get people to understand that it is better, cheaper, and faster than it used to be. There should not be a cloak of mystery on it anymore.
GV: Monte Carlo can be like having a hammer. The analyst must apply the controls. Monte Carlo does not have a built-in control.
How important is it to select the correct distribution for input parameters?
GV: It is only one part of the model. Get the model right first and then you can fine tune the model. The distribution should not drive the model.
JD: I agree with starting simple and building complexity. At the same time, you can’t “forget” to re-examine or improve the initial distribution.
GV: If you have some past data, use the distribution fitting that is in the software. There are few cases where you have all of the data you want or think you need. It is important to get started.
JD: Yes, get started and gain insights.
What is the most overlooked value of forecasting using Monte Carlo analysis?
JD: You do not have to have perfect data. Like what we were talking about, I can use the data I have and some expert elicitation to get a forecast and gain some insights. When we use single point estimates, we feel like we have to have perfect data, a lot of it, and it take a long time to get started.
GV: Mine is the value of correlations. Correlation creates the right tails in the model.
JD: Agree and getting the tails right is something other approaches can’t easily provide. It reminds me that Monte Carlo is a good complement to other methods. Although I prefer Monte Carlo as a primary tool, I often do not argue about it and simply use it as a triangulation tool for other forecast approaches.
GV: Monte Carlo is a good tool that provides good insights. One of its overlooked values is that it should help us think in ranges and help us to appreciate other approaches.
Both of you have worked in many different fields. What has surprised you most when it comes to forecasting?
GV: Many times simpler is better. Sometimes simpler is as much as you need. Even simpler than Monte Carlo.
JD: Most organizations do not use all of the data they have. They either don’t look for it or they think it’s not worth the effort.
GV: I have seen that in some cases people don’t have the time. Regretfully, sometimes they are just lazy.
JD: The other thing that has surprised me is the number of organizations that think they can just buy some software and good forecasts will just follow. This has generally gotten worse over the course of my career.
What is the best single tip that you would give someone who is preparing to lead a forecasting effort?
GV: Explore and understand the variables. Print it, graph it, and visualize it.
JD: I agree. Another key is to get enough different perspectives involved - finance, engineering, operations, maintenance, safety & security, and customer service. It helps us understand cause and effect as well as the different sources of the data that are available.
GV: One of the worst mistakes we have made is not getting the right people involved early. They got involved late, and we ended up being questioned on what we used some day and not others.
JD: I have had the same experience. Not just with data. Also, with methodology.
What else bothers you about forecasting in practice?
GV: So many people don’t do back-testing of the forecasts. It is really important to look back after a year and see how good the model is.
JD: I wholeheartedly agree. We don’t do back-testing and post-mortems enough. Sometimes we do not have the time or the funds to do so. Sometimes we just do not want to know.
GV: That’s right. We can’t get better if we don’t know if our forecasts were good or bad.
JD: We do not learn either. Or make better decisions.
Seth’s Sidebars
1. The biggest issue I have experienced is the ongoing confusion of correlation and causation.
2. The tendency is to throw out the forecasting effort as meaningless when the results deviate from the prediction.
3. The value of Monte Carlo analysis is getting away from THE result and focusing on the range of results.
4. For better or worse, most people probably know of Monte Carlo simulations through the popular writing of Nassim Taleb and their use in finance. Monte Carlo is bigger than that.
5. If you wait until you have all the information, it is too late. Better to get started but not hesitate making changes as more data is obtained.
Comments